![How Machine Learning Works for Cybersecurity [4 Ways]](/wp-content/uploads/2022/10/Importance-of-Machine-Learning-in-Cybersecurity.png)
Cybercrime is a problem affecting every industry and organization, but security isn’t just about protecting an organization from hackers. It also deals largely with preventing targeted attacks on data and intellectual property. And when it comes to online safety, machine learning in cybersecurity is a critical tool. As our understanding of cyber threats evolves, and technology continues advancing, so does the ability of machines to detect and respond.
In accomplishing this goal, it’s crucial to recognize how cyberattacks work. But it’s just as important to understand how machine learning can be used in OT cybersecurity projects. Artificial intelligence plays a vital role in many ways, such as detecting anomalies within data sets and identifying network weaknesses. So, let’s check out what machine learning in cybersecurity looks like and it’s importance to operational technology.
Understanding Machine Learning in Cybersecurity
Machine learning often describes making the process of having a computer “learn” by analyzing vast quantities of data. Then, it uses statistical techniques to make predictions and recommendations. As a matter of noteworthiness, machine learning algorithms can perform many various tasks. For example, facial recognition, spam detection, and even medical diagnosis.
Essentially, machine learning aims to create algorithms that can learn from and make predictions on data sets. This ultimately happens through training algorithms on a set of training data and validating it on a set of test data. The test data results can then help improve the overall machine learning model.
The success of any machine learning model depends on several factors. Data quality stands paramount since insufficient data leads to poor model performance. But machine learning models must also balance accuracy and interpretability, because too much of either can lead to problems. Furthermore, machine learning deployment platform must handle computational demands of the models, otherwise the models will not function correctly.
With all these factors in mind, model deployments can succeed. By paying attention to data quality, model design, and deployment platforms, organizations can set themselves up for success.
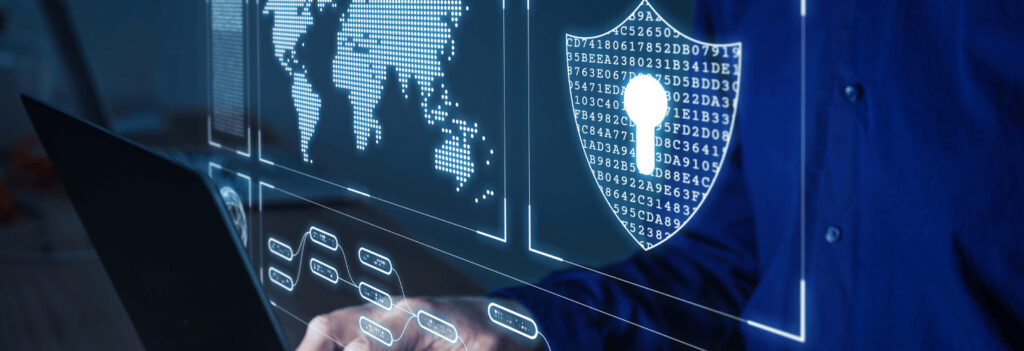
Machine Learning in Operational Technology Cybersecurity
Naturally, as the world becomes increasingly reliant on technology, the need for cybersecurity grows. A critical area of cybersecurity is operational technology (OT). Typically, critical infrastructure sectors such as energy, transportation, and manufacturing use operational technology systems. Furthermore, these systems are often complex and interconnected.
The operational technology cybersecurity guide provides a framework for organizations to assess and improve their cybersecurity posture. So, it’s a valuable resource for security professionals and those new to the field.
It covers various topics from risk management to incident response. And the creators regularly update it to reflect the latest threats and best practices. This makes it it an essential tool for anyone who wants to keep their organization safe from cyber attacks.
It’s important to note that machine learning doesn’t replace traditional approaches like threat monitoring. Instead, it helps automate these processes and improve them by providing more data points for analysis.
For example, if you want to detect suspicious activity on your network, you would use a variety of sensors. These might include firewalls, intrusion detection software (IDS), and machine learning tools such as anomaly detection engines (ADE).
The ADE then compares what it sees in real-time against known patterns within other systems (such as databases). Since it knows if something unusual is happening before anyone else, it helps organizations develop new ways to keep networks safe.
1. Detect Anomalies
One function of machine learning includes detecting anomalies in an operational technology network. By training a machine learning algorithm on a dataset of regular network activity, it can learn to identify standard behavior patterns.
When the algorithm is applied to new data, it can flag instances that deviate from the learned patterns as anomalous. So, this approach can help discover unusual traffic patterns, user behavior and other potentially malicious activity.
Machine learning can also improve the accuracy of automated detection tools by making them more effective at identifying threats. For example, some automated detection tools use “signature-based” algorithms that identify patterns in network traffic.
It alerts administrators when those patterns occur at particular times or during specific periods within a given day. These algorithms often come from statistical models trained with historical data from previous attacks.
2. Anti-Phishing
Historically, anti-phishing techniques have been based on external data, such as user reports. However, machine learning models can now extract key features from the same data that traditionally approaches use to identify malicious activity. Operational technology systems could use these features to detect suspicious patterns in events automatically.
3. Log Analysis
Machine learning is becoming increasingly popular in operational technology as the time it takes to analyze and troubleshoot data decreases. Log analysis and machine learning don’t represent entirely new concepts, but they have remained separate until now.
Machine learning can help reduce this time by providing a solution to log analysis based on analyzing data from multiple sources. Machine-learning algorithms can find patters in a large amount of data, making them more effective than traditional log analysis techniques.
Using machine learning to secure operational technology systems can help protect critical infrastructure and keep the world running smoothly.
4. Future Prediction
Machine learning is a field that has gained more and more popularity. The main reason behind this is that it can do many things, like future prediction, which operational technology in cybersecurity needs.
In the operational technology, there exists much past and present data about the behavior of the systems while dealing with a cyberattack. We can use this data to build a model which makes future predictions.
This sounds like an excellent idea for operational technology in cybersecurity because we know how hackers act from experience. So, we can use that knowledge to predict what they will do next time they hack into a system.
Since so many cyber incidents happen daily, an automated system can quickly detect patterns among them. It will make it easier for security teams worldwide to prevent future attacks.
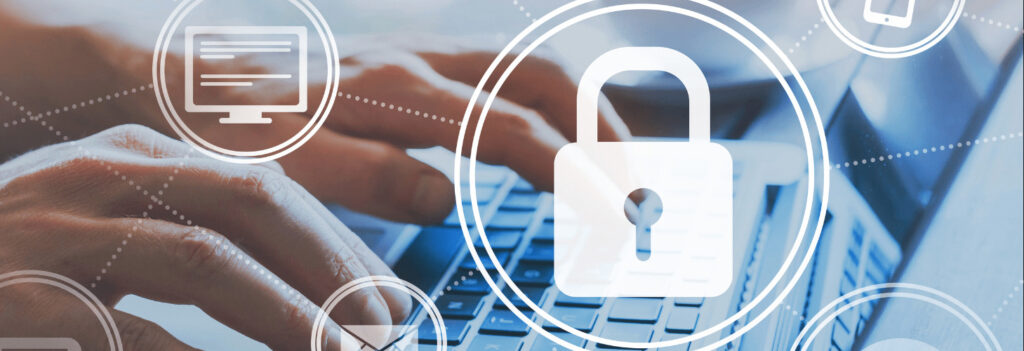
Final Thoughts On Machine Learning in Cybersecurity
In conclusion, machine learning has the potential to become an invaluable tool in cybersecurity. Using machine learning algorithms, systems can train to detect anomalies or patterns that may indicate a threat or malicious activity.
The ability of these algorithms to adapt and learn from new data sets will likely make them increasingly valuable as time goes on. However, some challenges still need to be overcome before we see widespread use in operational technology cybersecurity.
Firstly, most operational technologies do not have access to the equipment, such as high-performance computers required for advanced analytics. Secondly, companies need better training programs for their employees to know how best to apply this technology.
Last Updated:
Article guest written by Daniel Martin. Dan has hands-on experience in digital marketing as far back as 2007. He has been building teams and coaching others to foster innovation and solve real-time problems. Dan also enjoys photography and traveling.